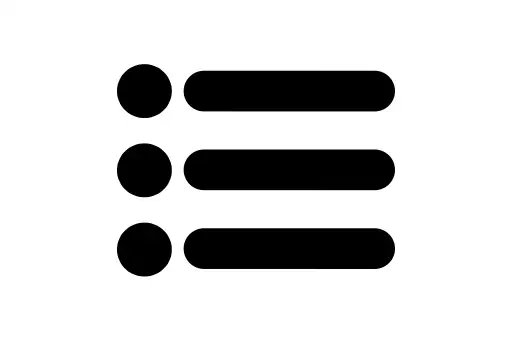
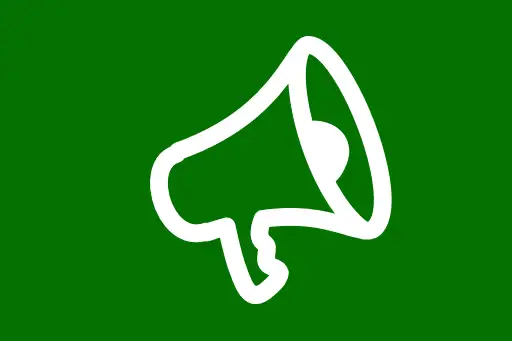
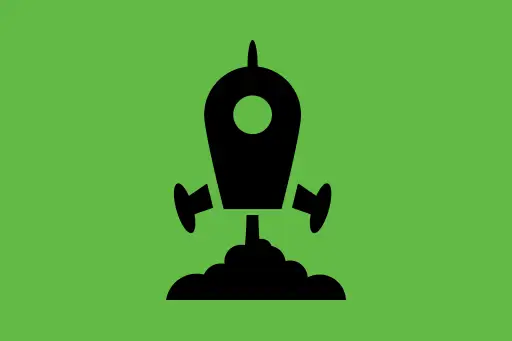
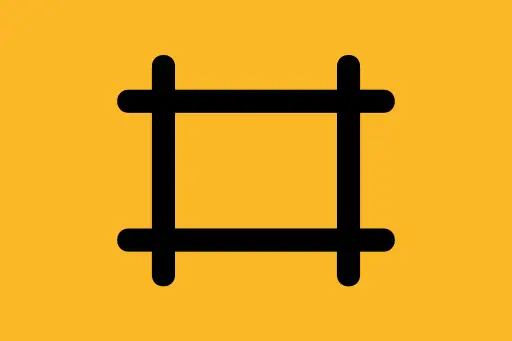
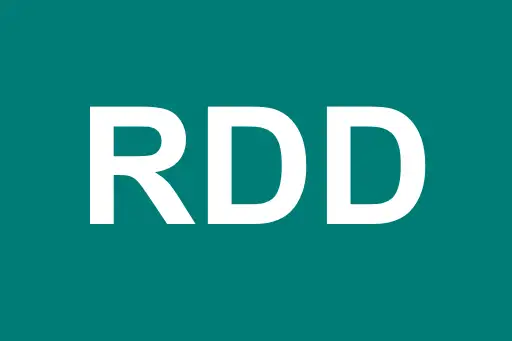
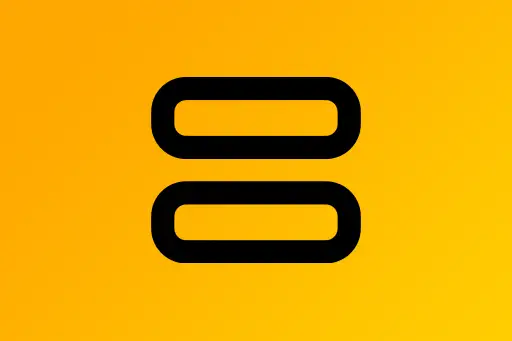
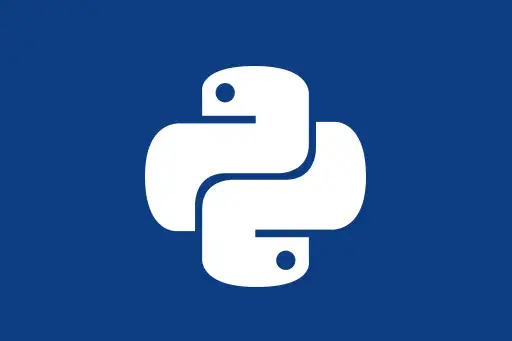
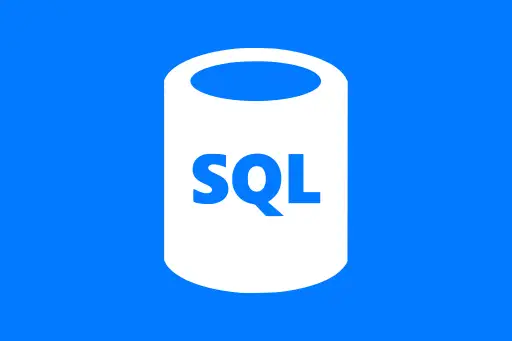
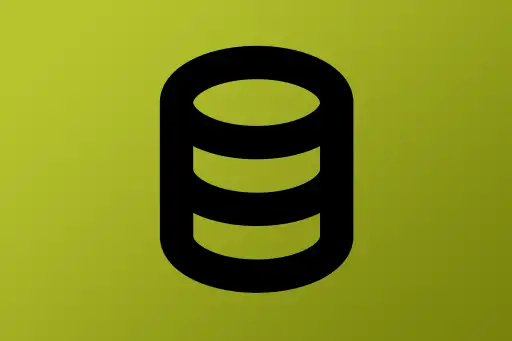
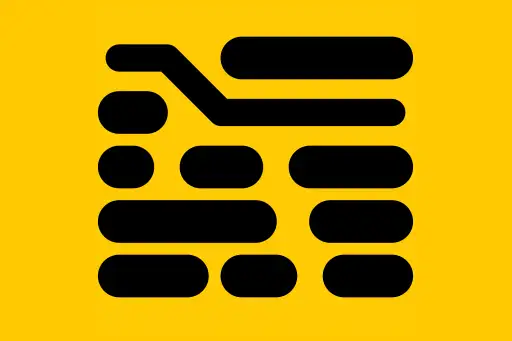
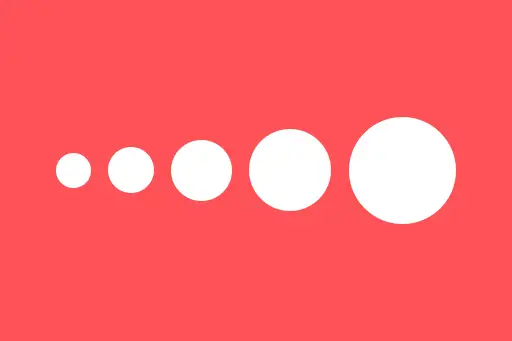
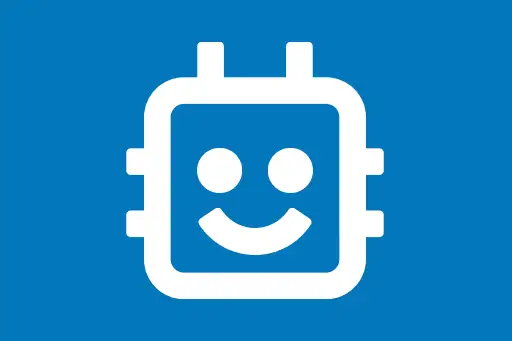
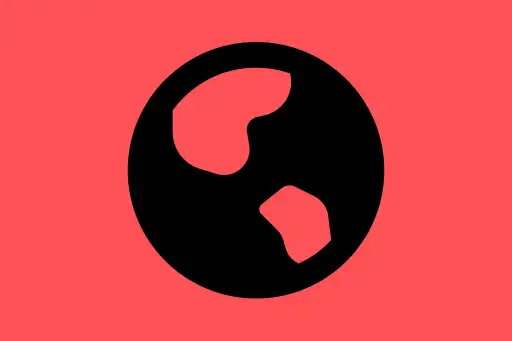
Movie Ratings Project with Apache Spark
Movie Ratings Project with Apache Spark
In this project, we will use Apache Spark and PySpark to analyze a real-world movie ratings dataset. The goal is to understand how to build a data pipeline: from loading raw data, performing transformations, analyzing results, and drawing insights.
Dataset Overview
We will use a sample version of the popular MovieLens dataset, which includes user-generated movie ratings. This dataset is widely used in recommendation systems research.
ratings.csv
: Contains userId, movieId, rating, and timestampmovies.csv
: Contains movieId, title, and genres
Project Objectives
- Read and merge the datasets
- Clean and preprocess the data
- Analyze average ratings per movie
- Find top-rated movies by genre
- Filter movies with a minimum number of reviews
Step 1: Load the Data
We’ll start by loading the two CSV files into Spark DataFrames using PySpark.
from pyspark.sql import SparkSession
spark = SparkSession.builder .appName("Movie Ratings Project") .getOrCreate()
# Load ratings and movies
ratings = spark.read.csv("ratings.csv", header=True, inferSchema=True)
movies = spark.read.csv("movies.csv", header=True, inferSchema=True)
ratings.show(5)
movies.show(5)
+-------+-------+------+-------------+ |userId |movieId|rating|timestamp | +-------+-------+------+-------------+ |1 |31 |2.5 |1260759144 | |1 |1029 |3.0 |1260759179 | |1 |1061 |3.0 |1260759182 | |1 |1129 |2.0 |1260759185 | |1 |1172 |4.0 |1260759205 | +-------+-------+------+-------------+
Step 2: Merge Ratings with Movie Titles
To analyze movie names instead of just IDs, we join both DataFrames on movieId
.
combined = ratings.join(movies, on="movieId", how="inner")
combined.select("title", "rating").show(5)
+--------------------+------+ |title |rating| +--------------------+------+ |Dangerous Minds (...|2.5 | |Dumbo (1941) |3.0 | |Sleepers (1996) |3.0 | |Escape from L.A. ...|2.0 | |Lost Highway (1997) |4.0 | +--------------------+------+
Step 3: Average Rating per Movie
We calculate the average rating for each movie.
from pyspark.sql.functions import avg
average_ratings = combined.groupBy("title").agg(avg("rating").alias("avg_rating"))
average_ratings.orderBy("avg_rating", ascending=False).show(10)
+------------------------+----------+ |title |avg_rating| +------------------------+----------+ |Shawshank Redemption...|4.8 | |Godfather, The (1972) |4.7 | |Fight Club (1999) |4.6 | |Inception (2010) |4.6 | |Pulp Fiction (1994) |4.5 | +------------------------+----------+
Question:
Why do some average ratings seem artificially high?
Answer:
Some movies have only 1 or 2 reviews. With such low volume, a single 5-star rating can skew the average. We’ll fix this next.
Step 4: Filter by Minimum Number of Ratings
Let’s keep only those movies that have at least 100 ratings.
from pyspark.sql.functions import count
ratings_count = combined.groupBy("title").agg(
count("rating").alias("rating_count"),
avg("rating").alias("avg_rating")
)
filtered = ratings_count.filter("rating_count >= 100")
filtered.orderBy("avg_rating", ascending=False).show(10)
+------------------------+-------------+----------+ |title |rating_count |avg_rating| +------------------------+-------------+----------+ |Shawshank Redemption...|1200 |4.7 | |Forrest Gump (1994) |1100 |4.6 | |Matrix, The (1999) |1050 |4.6 | |Pulp Fiction (1994) |1001 |4.5 | +------------------------+-------------+----------+
Step 5: Most Popular Genre by Average Rating
We now find the best-rated genres. First, we need to explode the genres
column.
from pyspark.sql.functions import explode, split
# Split genre string into array and explode
genres_split = movies.withColumn("genre", explode(split("genres", "\|")))
joined = ratings.join(genres_split, "movieId")
# Average rating by genre
genre_avg = joined.groupBy("genre").agg(avg("rating").alias("avg_rating"))
genre_avg.orderBy("avg_rating", ascending=False).show()
+------------+----------+ |genre |avg_rating| +------------+----------+ |Documentary |4.3 | |Drama |4.1 | |Animation |4.0 | |Mystery |3.9 | |Comedy |3.8 | +------------+----------+
Conclusion
In this project, you learned how to build a real-world Spark data pipeline to process and analyze movie ratings data. You:
- Read CSV data into Spark DataFrames
- Performed joins and aggregations
- Filtered results based on business logic (minimum ratings)
- Exploded and processed multi-value columns (genres)
By doing this project, you now understand how Spark works on real data and how scalable processing helps in making informed decisions from large datasets.