- 1Machine Learning – Loading Datasets in Python (CSV, Excel, Built-in, and URLs)
- 2Handling Missing Values and Outliers in Machine Learning
- 3Encoding Categorical Data in Machine Learning (Beginner-Friendly Guide)
- 4Machine Learning - Feature Scaling and Normalization
- 5Train-Test Split in Machine Learning (With Examples and Python Code)
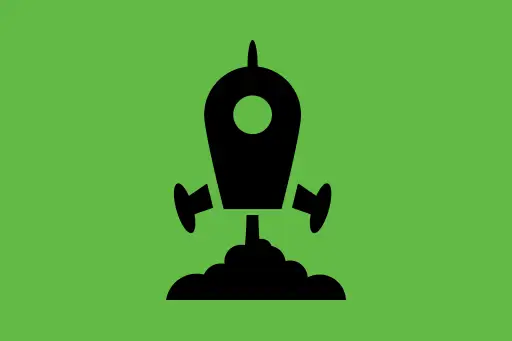
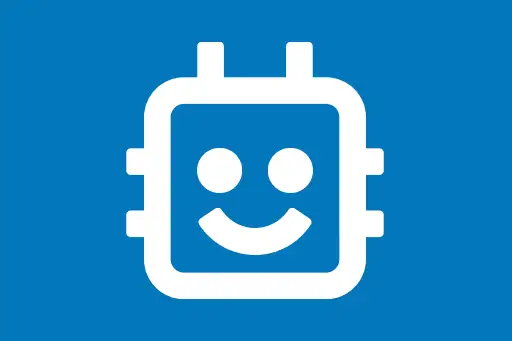
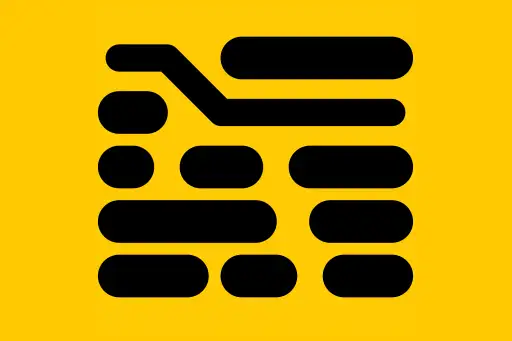
- 1Machine Learning – Loading Datasets in Python (CSV, Excel, Built-in, and URLs)
- 2Handling Missing Values and Outliers in Machine Learning
- 3Encoding Categorical Data in Machine Learning (Beginner-Friendly Guide)
- 4Machine Learning - Feature Scaling and Normalization
- 5Train-Test Split in Machine Learning (With Examples and Python Code)
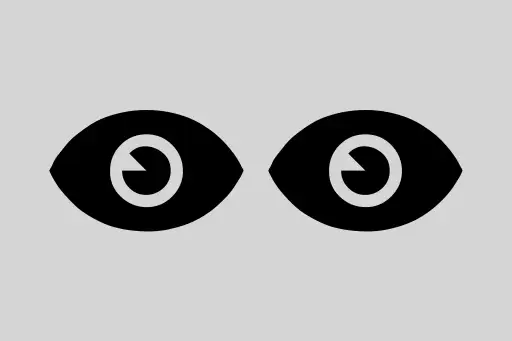
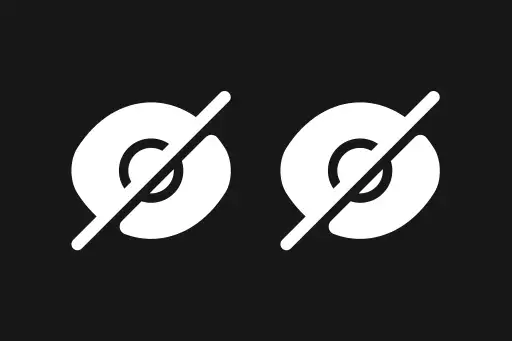
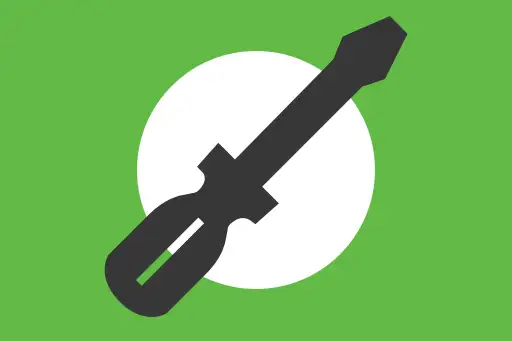
Handling Missing Values and Outliers in Machine Learning
Next Topic ⮕Encoding Categorical Data in Machine Learning (Beginner-Friendly Guide)
Handling Missing Values and Outliers in Machine Learning
Before we feed any dataset to a machine learning model, we must clean and prepare the data. Two of the most common issues you’ll face during data cleaning are:
- Missing Values – when some data entries are blank or not available
- Outliers – data points that are significantly different from the rest of the data
Part 1: Handling Missing Values
Missing values can cause issues with ML models since most models do not accept data with missing entries. Let's look at why missing values occur and how to deal with them.
Why do missing values occur?
- Human error in data entry
- Sensor failures (e.g., weather station didn’t record temperature)
- Participants skipped a question in a survey
Example Dataset
Suppose we are building a model to predict house prices and the dataset looks like this:
House ID | Location | Size (sqft) | Bedrooms | Price (in $) |
---|---|---|---|---|
1 | New York | 1000 | 2 | 500000 |
2 | Los Angeles | NaN | 3 | 650000 |
3 | NaN | 1500 | NaN | 700000 |
4 | Chicago | 1200 | 2 | NaN |
We have missing values in Location
, Size
, Bedrooms
, and Price
.
Common Strategies to Handle Missing Values
- Drop rows or columns
- Drop rows where important values are missing
- Drop entire columns if they are mostly empty
- Imputation
- Numerical: Replace with mean, median, or mode
- Categorical: Replace with most frequent value
Question:
Why not always drop rows with missing values?
Answer:
Dropping too many rows can lead to data loss and bias. It's best only if very few rows have missing values or the row contains little useful information.
Python Code – Handling Missing Values
import pandas as pd
import numpy as np
# Sample data
data = {
'House ID': [1, 2, 3, 4],
'Location': ['New York', 'Los Angeles', np.nan, 'Chicago'],
'Size (sqft)': [1000, np.nan, 1500, 1200],
'Bedrooms': [2, 3, np.nan, 2],
'Price': [500000, 650000, 700000, np.nan]
}
df = pd.DataFrame(data)
# Show original dataset
print("Original Data:")
print(df)
# Fill missing numerical values with mean
df['Size (sqft)'].fillna(df['Size (sqft)'].mean(), inplace=True)
df['Bedrooms'].fillna(df['Bedrooms'].mode()[0], inplace=True)
df['Price'].fillna(df['Price'].median(), inplace=True)
# Fill missing categorical value with mode
df['Location'].fillna(df['Location'].mode()[0], inplace=True)
# Final cleaned data
print("\nCleaned Data:")
print(df)
Code Explanation:
fillna()
is used to replace missing values.- We use:
- mean for
Size
- mode for
Bedrooms
(most common value) - median for
Price
to reduce the impact of outliers - mode for
Location
(categorical)
- mean for
Part 2: Handling Outliers
Outliers are data points that are significantly different from the rest. For example, in a dataset of house prices ranging from 300K to 800K, a price of 5 million is an outlier.
How do outliers affect ML models?
- Can skew mean and standard deviation
- Can reduce model accuracy and increase error
How to detect outliers?
- Boxplot – visually shows data distribution
- Z-score method – values beyond 3 standard deviations
- IQR method – values below Q1 - 1.5*IQR or above Q3 + 1.5*IQR
Python Code – Detect and Remove Outliers using IQR
import matplotlib.pyplot as plt
# Boxplot to visualize outliers
plt.boxplot(df['Price'])
plt.title("Boxplot for Price")
plt.show()
# Detecting outliers using IQR
Q1 = df['Price'].quantile(0.25)
Q3 = df['Price'].quantile(0.75)
IQR = Q3 - Q1
# Filtering data
lower_bound = Q1 - 1.5 * IQR
upper_bound = Q3 + 1.5 * IQR
df_no_outliers = df[(df['Price'] >= lower_bound) & (df['Price'] <= upper_bound)]
print("Data without outliers:")
print(df_no_outliers)
Code Explanation:
quantile()
gets the 25th (Q1) and 75th (Q3) percentiles.IQR
is the Interquartile Range = Q3 - Q1- We calculate lower and upper bounds to filter out data outside the IQR range.
Question:
Why use median instead of mean when handling outliers?
Answer:
The median is less affected by outliers and better represents the center of skewed data, making it more reliable for imputation or central tendency in such cases.
Summary
- Missing Values can be handled using drop or imputation (mean/median/mode)
- Outliers can be detected using boxplots, z-scores, or IQR and should be carefully removed or capped
- Clean data ensures better model accuracy and generalization
Handling data quality is one of the most important skills in a machine learning project, even before the algorithm selection. The better the data, the better the model!